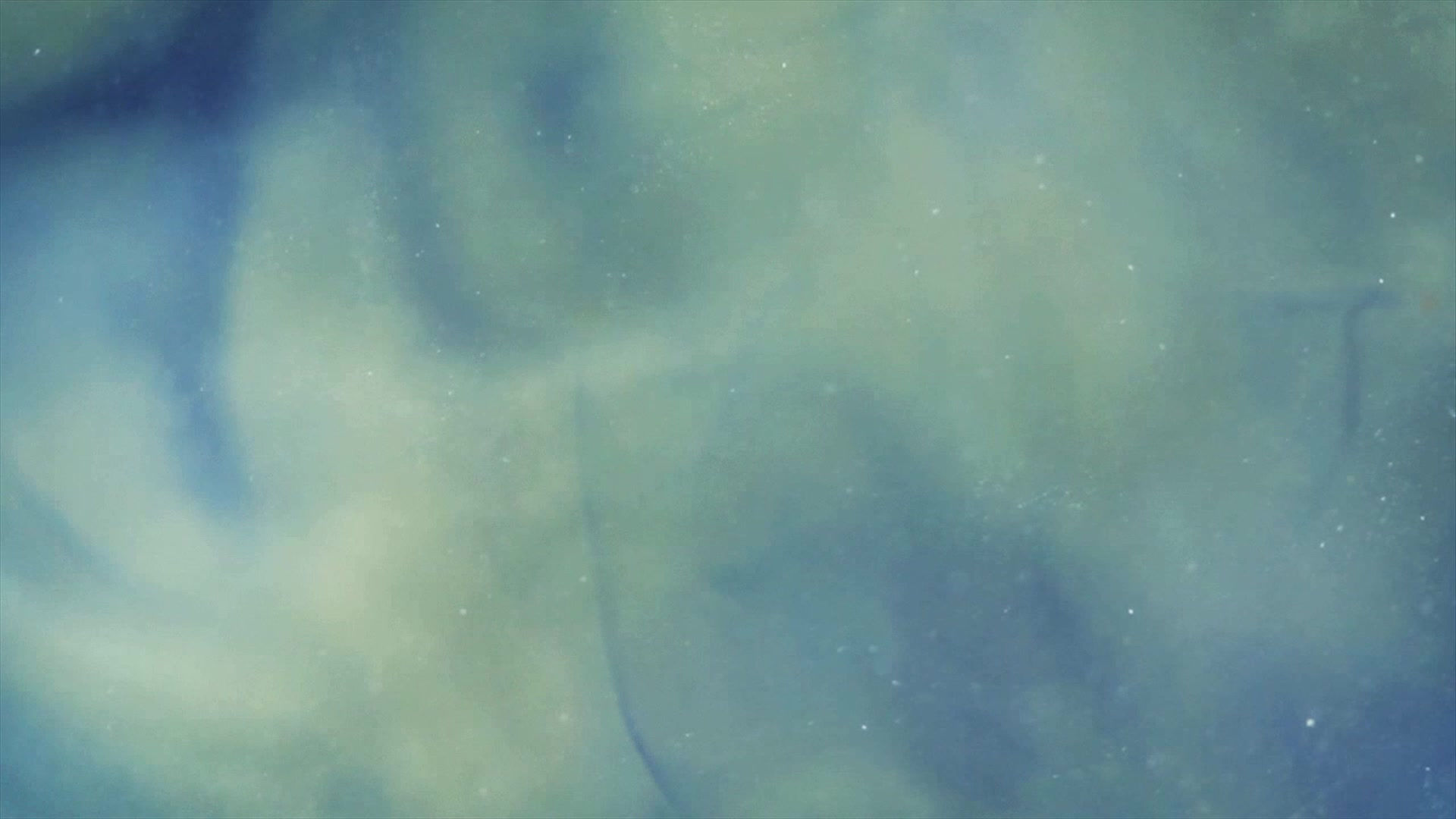
Haibin Di, Ph.D.
PUBLICATIONS
-
Di, H., N. Pham, and A. Abubakar, 2024, Estimating geotechnical parameters using semi-supervised learning: An example to the Dutch offshore wind farm zone: Geophysics, 89(1): WA85-WA93. (Link) (PDF)
-
Di, H., W. Hu, A. Abubakar, P. Devarakota, W. Li, and Y. Li, 2024, Latest advancements in machine learning for geophysics – Introduction: Geophysics, 89(1): 1-4. (Link) (PDF)
-
Waheed, U. B., H. Di, J. Sun, and D. Angus, 2024, Introduction to special issue on machine learning applications in geophysical exploration and monitoring: Geophysical Prospecting, 72(1): 3-6. (Link) (PDF)
-
Di, H., and A. Abubakar, 2023, Automating seismic-well tie via self-supervised learning: Geophysical Prospecting, 71(4): 698-712. (Link) (PDF)
-
Di, H., and A. Abubakar, 2022, Automated active learning in seismic image interpretation: The Leading Edge, 41(9): 628-635. (Link) (PDF)
-
Abubakar, A., H. Di, A. Kaul, C. Li, Z. Li, V. Simoes, L. Truelove, and T. Zhao, 2022, Deep learning for end-to-end subsurface modeling and interpretation: An example from the Groningen gas field: The Leading Edge, 41(4): 259-267. (Link) (PDF)
-
Di, H., Z. Li, and A. Abubakar, 2022, Using relative geologic time to constrain convolutional neural network-based seismic interpretation and property estimation: Geophysics, 87(2): IM25-IM35. (Link) (PDF)
-
Di, H., and A. Abubakar, 2022, Estimating subsurface properties using a semisupervised neural network approach: Geophysics, 87(1): IM1-IM10. (Link) (PDF)
-
Abriel, B., M. Araya-Polo, H. Di, S. Fomel, J. Lomask, P. Nivlet, J. Vamaraju, and B. Wallet, 2022, Introduction to special section: Automated approaches to interpretation: Interpretation, 10(2): Sci-Sci. (Link) (PDF)
-
Shafiq, M. A., Z. Long, H. Di, and G. AlRegib, 2021, A novel attention model for salient structure detection in seismic volumes: Applied Computing and Intelligence, 1(1), 31-45. (Link) (PDF)
-
Di, H., C. Li, S. Smith, Z. Li, and A. Abubakar, 2021, Imposing interpretational constraints on a seismic interpretation convolutional neural network: Geophysics, 86(3), IM63-IM71. (Link) (PDF)
-
Di, H., A. Kaul, L. Truelove, W. Li, W. Hu, and A. Abubakar, 2021, Data Analytics and Machine Learning Hackathon 2021: A deep dive into the open-source data challenge for E&P: The Leading Edge, 40(1), 68-71. (Link) (PDF)
-
Di, H., L. Truelove, C. Li, and A. Abubakar, 2020, Accelerating seismic fault and stratigraphy interpretation with deep CNNs: A case study of the Taranaki Basin, New Zealand: The Leading Edge, 39(10), 727-733. (Link) (PDF)
-
Di, H., Z. Li, H. Maniar, and A. Abubakar, 2020, Seismic stratigraphy interpretation by deep convolutional neural networks: A semisupervised workflow: Geophysics, 85(4), WA77-WA86. (Link) (PDF)
-
Di, H., and G. AlRegib, 2020, A comparison of seismic saltbody interpretation via neural networks at sample and pattern levels: Geophysical Prospecting, 68(2), 521-535. (Link) (PDF)
-
Di, H., M. A. Shafiq, Z. Wang, and G. AlRegib, 2019, Improving seismic fault detection by super-attribute-based classification: Interpretation, 7(3), SE251-SE267. (Link) (PDF)
-
Di, H., D. Gao, and G. AlRegib, 2019, Developing a seismic texture analysis neural network for machine-aided seismic pattern recognition and classification: Geophysical Journal International, 218(2), 1262-1275. (Link) (PDF)
-
Di, H., and G. AlRegib, 2019, Semiautomatic fault/fracture interpretation based on seismic geometry analysis: Geophysical Prospecting, 67(5), 1379-1391. (Link) (PDF)
-
Di, H., M. Alfarraj, and G. AlRegib, 2019, Three‐dimensional curvature analysis of seismic waveforms and its interpretational implications: Geophysical Prospecting, 67(2), 265-281. (Link) (PDF)
-
Di, H., and G. AlRegib, 2019, Reflector dip estimates based on seismic waveform curvature/flexure analysis: Interpretation, 7(2), SC1-SC9. (Link) (PDF)
-
Di, H., T. Zhao, V. Jayaram, X. Wu, L. Huang, G. AlRegib, J. Cao, M. Araya-Polo, S. Chopra, S. Al-Dossary, F. Li, E. Gloaguen, Y. Lin, A. Solberg, and H. Zeng, 2019, Introduction to special section: Machine learning in seismic data analysis: Interpretation, 7(3); Sci-SCii. (Link) (PDF)
-
Wu, X., H. Zeng, H. Di, D. Gao, J. Gao, K. Marfurt, S. al Dossary, and G. Dorn, 2019, Introduction to special section: Seismic geometric attributes: Interpretation, 7(2): Sci-Sci. (Link) (PDF)
-
Payam, K., T. Wilson, T. Carr, A. Kumar, R. Hammack, and H. Di, 2019, Integrating distributed acoustic sensing (DAS) and borehole 3C geophone array data to identify long-period long-duration seismic events during stimulation of a Marcellus shale gas reservoir: Interpretation, 7(1), SA1-SA10. (Link) (PDF)
-
Di, H., M. A. Shafiq, and G. AlRegib, 2018, Multi-attribute k-means clustering for salt boundary delineation from 3D seismic data: Geophysical Journal International, 215(3), 1999-2007. (Link) (PDF)
-
Di, H., D. Gao, and G. AlRegib, 2018, 3D dip vector-guided auto-tracking for weak seismic reflections: A new tool for shale reservoir visualization and interpretation: Interpretation, 6(4), SN47-SN56. (Link) (PDF)
-
Long, Z., Y. Alaudah, M.A. Qureshi, Y. Hu, Z. Wang, M. Alfarraj, G. AlRegib, A. Amin, M. Deriche, S. Al-Dharrab, and H. Di, 2018, A comparative study of texture attributes for characterizing subsurface structures in seismic volumes: Interpretation, 6(4), T1055-T1066. (Link) (PDF)
-
Shafiq, M. A., H. Di, and G. AlRegib, 2018, A novel approach for automated detection of listric faults within migrated seismic volumes: Journal of Applied Geophysics, 155, 94-101. (Link) (PDF)
-
Wang, Z., H. Di, M. A. Shafiq, Y. Alaudah, and G. AlRegib, 2018, Successful leveraging of image processing and machine learning in seismic structural interpretation: A review: The Leading Edge, 37(6), 451-461. (Link) (PDF)
-
AlRegib, H., M. Deriche, Z. Long, H. Di, Z. Wang, Y. Alaudah, M. A. Shafiq, M. Alfarraj, 2017, Subsurface Structure Analysis using Computational Interpretation and Learning: A Visual Signal Processing Perspective: IEEE Signal Processing Magazine, 35(2), 82-98. (Link) (PDF)
-
Di, H., and D. Gao, 2017, Nonlinear gray-level co-occurrence matrix texture analysis for improved seismic facies interpretation: Interpretation, 5(3), SJ31-SJ40. (Link) (PDF)
-
Di, H., and D. Gao, 2017, 3D seismic flexure analysis for subsurface fault detection and fracture characterization: Pure and Applied Geophysics, 174(3), 747-761. (Link) (PDF)
-
Huang, Y., H. Di, R. Malekian, X. Qi, and Z. Li, 2017, Noncontact measurement and detection of instantaneous seismic attributes based on complementary ensemble empirical mode decomposition: Energies, 10(10), 1655. (Link) (PDF)
-
Di, H., and D. Gao, 2016, Improved estimates of seismic curvature and flexure based on 3D surface rotation in the presence of structure dip: Geophysics, 81(2), IM37-IM47. (Link) (PDF)
-
Di, H., and D. Gao, 2016, Efficient volumetric extraction of most positive/negative curvature and flexure attributes for improved fracture characterization from 3D seismic data: Geophysical Prospecting, 64(6), 1454-1468. (Link) (PDF)
-
Gao, D., and H. Di, 2015, Extreme curvature and extreme flexure analysis for fracture characterization from 3D seismic data: New analytical workflows and geologic implications: Geophysics, 80(2), IM11-IM20. (Link) (PDF)
-
Di, H., and D. Gao, 2014, Gray-level transformation and Canny edge detection for 3D seismic discontinuity enhancement: Computers & Geosciences, 72, 192-200. (Link) (PDF)
-
Di, H., and D. Gao, 2014, A new algorithm for evaluating 3D curvature and curvature gradient for improved fracture detection: Computers & Geosciences, 70, 15-25. (Link) (PDF)
-
Zheng, Z., P. Kavousi, and H. Di, 2014, Multi-attribute and neural network-based fault detection in 3D seismic interpretation: Advanced Materials Research, 838-841, 1497-1502. (Link) (PDF)
-
邸海滨, 许力生, 2012, 层状介质中有限震源引起的地面运动的计算——对点源情形的拓展: 地震学报, 34(4), 425-438. (Link) (PDF) Citation in English: Di, H., and L. Xu, 2012, Calculation of the ground motion generated by a finite source in stratified media: An extension of the point-source case: Acta Seismologica Sinica (in Chinese), 34(4), 425-438.
-
邸海滨, 郭玉倩, 刘喜武, 2011, 基于稀疏约束贝叶斯估计的相对波阻抗反演: 石油物探, 50(2), 124-128. (Link) (PDF) Citation in English: Di, H., Y. Guo, and X.W. Liu, 2011, The inversion algorithm of seismic relative acoustic impedance based on Cauchy sparseness constraint Bayesian estimation: Geophysical Prospecting for Petroleum (in Chinese), 50(2), 124-128.
-
许力生, 邸海滨, 冯万鹏, 李春来, 2010, 2010年青海玉树Ms 7.1地震近断层地面运动估计: 地球物理学报, 53(6), 1366-1373. (Link) (PDF) Citation in English: Xu, L., H. Di, W. Feng, et al, 2010, Estimation of the fault-near ground motion of the 2010 Yushu, Qinghai, Ms7.1 earthquake: Chinese J. Geophys. (in Chinese), 53(6),1366-1373.
Journal Papers


Conference (Expanded) Abstracts
-
Di, H., and A. Abubakar, 2023, Stochastic windfarm soil property estimation via deep CNNs: 4th EAGE Global Energy Transition Conference and Exhibition, 1-5. (Link) (PDF)
-
Dhar Gupta, K., H. Di, and A. Abubakar, 2023, Automatic estimation of reservoir properties using 3D machine learning workflow independent of well alignment in the presence of geological complexes: Abu Dhabi International Petroleum Exhibition & Conference, SPE-216867-MS. (Link) (PDF)
-
Di, H., and A. Abubakar, 2023, Structure-constrained windfarm soil property estimation via deep neural networks: 84th EAGE Annual Conference and Exhibition, 1-5. (Link) (PDF)
-
Di, H., and A. Abubakar, 2023, Estimating elastic properties from angle-stack seismic data via deep neural networks: 84th EAGE Annual Conference and Exhibition, 1-5. (Link) (PDF)
-
Shao, T., W. Hu, S. Phan, H. Di, and A. Abubakar, 2023, Time-lapse seismic data deblending with deep learning: 84th EAGE Annual Conference and Exhibition, 1-5. (Link) (PDF)
-
Zhao, T., H. Di, and A. Abubakar, 2023, Cascaded deep learning for offshore wind farm 2D seismic horizon interpretation: 84th EAGE Annual Conference and Exhibition, 1-5. (Link) (PDF)
-
Di, H., and A. Abubakar, 2023, An integrated workflow for windfarm site characterization by deep learning: 3rd EAGE Digitalization Conference and Exhibition, 1-6. (Link) (PDF)
-
Abubakar, A., H. Di, S. Manikani, and T. Zhao, 2023, How deep learning can accelerate offshore wind farm site characterization: AAPG Explorer. (Link)
-
Di, H., and A. Abubakar, 2022, Accelerating geotechnical soil characterization in offshore windfarm sites via semi-supervised learning: 3rd EAGE Global Energy Transition Conference & Exhibition. (Link) (PDF)
-
Di, H., and A. Abubakar, 2022, Self-Supervised learning for automated seismic wavelet extraction: Abu Dhabi International Petroleum Exhibition & Conference, SPE-211823-MS. (Link) (PDF)
-
Di, H., and A. Abubakar, 2022, Semi-supervised learning for geotechnical soil property estimation in offshore windfarm sites: Abu Dhabi International Petroleum Exhibition & Conference, SPE-211836-MS. (Link) (PDF)
-
Di., H., V. Simoes, Z. Li, C. Li, A. Kaul, and A. Abubakar, 2022, An integrated workflow for deep learning-accelerated seismic modeling of the Groningen gas field, the Netherlands: 92nd SEG Technical Program Expanded Abstracts, 1805-1809. (Link) (PDF)
-
Zhao, T., H. Di, and A. Abubakar, 2022, Validating machine learning-based seismic property prediction through self-supervised seismic reconstruction: 92nd SEG Technical Program Expanded Abstracts, 1800-1804. (Link) (PDF)
-
Di, H., L. Truelove, and A. Abubakar, 2022, Automated active learning for seismic facies classification: 92nd SEG Technical Program Expanded Abstracts, 1694-1698. (Link) (PDF)
-
Di, H., A. Abubakar, 2022, Unsupervised learning for automated seismic-well tie: 83rd EAGE Annual Conference and Exhibition. (Link) (PDF)
-
K. Dhar Gupta, H. Di, and A. Abubakar, 2022, Improving automatic estimation of rock properties in the presence of geological complexes using machine learning: 83rd EAGE Annual Conference and Exhibition. (Link) (PDF)
-
Di, H., C. K. Kloucha, C. Li, A. Abubakar, Z. Li, H. B. Jeddou, and H. Mustapha, Fault-guided seismic stratigraphy interpretation via semi-supervised learning: Abu Dhabi International Petroleum Exhibition & Conference, SPE-207218-MS. (Link) (PDF)
-
Di, H., Z. Li, and A. Abubakar, 2021, Using relative geologic time to constrain seismic facies classification using neural networks: 91st SEG Technical Program Expanded Abstracts, 991-995. (Link) (PDF)
-
Abubakar, A., M. J. Brædstrup, H. Di, A. T. Diaz, S. Freeman, S. Hviid, K. H. Karkov, S. Kriplani, S. Manikani, G. Salun, and T. Zhao, Deep learning applications for wind farms site characterization and monitoring: 91st SEG Technical Program Expanded Abstracts, 3009-3013. (Link) (PDF)
-
Zhao, T., B. Sankaranarayanan, H. Di, and A, Abubakar, Using backward models to validate the goodness-of-fit in machine learning predictions: 91st SEG Technical Program Expanded Abstracts, 1435-1439. (Link) (PDF)
-
Di, H., and A. Abubakar, 2021, Using semi-supervised convolutional neural networks for porosity modeling over a fluvio-deltaic Triassic gas field: SPE Annual Technical Conference and Exhibition, SPE-205841-MS. (Link) (PDF)
-
Cai, A., H. Di, Z. Li, H. Maniar, and A. Abubakar, 2020, Wasserstein cycle-consistent generative adversarial network for improved seismic impedance inversion: Example on 3D SEAM model: 90th SEG Technical Program Expanded Abstracts, 1274-1278. (Link) (PDF)
-
Di, H., X. Chen, H. Maniar, and A. Abubakar, 2020, Semi-supervised seismic and well log integration for reservoir property estimation: 90th SEG Technical Program Expanded Abstracts, 2166-2170. (Link) (PDF)
-
Guo, R., H. Maniar, H. Di, N. Moldoveanu, A. Abubakar, and M. Li, 2020, Ground roll attenuation with an unsupervised deep learning approach: 90th SEG Technical Program Expanded Abstracts, 3164-3168. (Link) (PDF)
-
Bhattacharya, S., and H. Di, 2020, The classification and interpretation of the polyphase fault network on the North Slope, Alaska using deep learning: 90th SEG Technical Program Expanded Abstracts, 3847-3851. (Link) (PDF)
-
Di, H., X. Chen, H. Maniar, and A. Abubakar, 2020, A semi-supervised learning framework for seismic acoustic impedance estimation: EAGE/AAPG Digital Subsurface for Asia Pacific Conference, 1-3 (Link) (PDF)
-
Di, H., C. Li, S. Smith, and A. Abubakar, 2019, Machine learning-assisted seismic interpretation with geologic constraints: 89th SEG Technical Program Expanded Abstracts, 5360-5364. (Link) (PDF)
-
Di, H., Z. Li, H. Maniar, and A. Abubakar, 2019, Seismic stratigraphy interpretation via deep convolutional neural networks: 89th SEG Technical Program Expanded Abstracts, 2358-2362. (Link) (PDF)
-
Li, Z., H. Di, H. Maniar, and A. Abubakar, 2019, Semi-supervised deep machine learning assisted seismic image segmentation and stratigraphic sequence interpretation: 81st EAGE Conference & Exhibition, Th_P04_04. (Link) (PDF)
-
Di, H., Z. Wang, and G, AlRegib, 2018, Real-time seismic image interpretation via deconvolutional neural network: 88th SEG Technical Program Extended Abstracts, 2051-2055. (Link) (PDF)
-
Di, H., Z. Wang, and G, AlRegib, 2018, Why using CNN for seismic interpretation? An investigation: 88th SEG Technical Program Extended Abstracts, 2216-2220. (Link) (PDF)
-
Di, H., Shafiq, M., and G. AlRegib, 2018, Patch-level MLP classification for improved fault detection: 88th SEG Technical Program Extended Abstracts, 2211-2215. (Link) (PDF)
-
Shafiq, M., M. Prabhushankar, H. Di, and G. AlRegib, 2018, Towards understanding common features between natural and seismic images: 88th SEG Technical Program Extended Abstracts, 2076-2080. (Link) (PDF)
-
Di, H., Z. Wang, and G. AlRegib, 2018, Seismic fault detection from post-stack amplitude by convolutional neural networks: 80th EAGE Conference and Exhibition, Tu-D-11. (Link) (PDF) (Slides)
-
Di, H., Z. Wang, and G. AlRegib, 2018, Deep convolutional neural networks for seismic salt-body delineation: AAPG Annual Convention and Exhibition, Search and Discovery Article #90323. (Link)
-
Shafiq, M., Z. Long, H. Di, G. AlRegib, and M. Deriche, 2018, Fault detection using attention models based on visual saliency: ICASSP 2018. (Link)
-
Di, H., and G. AlRegib, 2017, A new method for dip estimation based on seismic waveform curvature/flexure analysis: 87th SEG Technical Program Extended Abstracts, 2260-2264. (Link) (PDF) (Slides)
-
Di, H., M. Alfarraj, and G. AlRegib, 2017, 3D curvature analysis of seismic waveform and its interpretational implications: 87th SEG Technical Program Extended Abstracts, 2255-2259. (Link) (PDF) (Slides)
-
Di, H., M. A. Shafiq, and G. AlRegib, 2017, Seismic fault detection based on multi-attribute support vector machine analysis: 87th SEG Technical Program Extended Abstracts, 2039-2044. (Link) (PDF) (Slides)
-
Alfarraj, M., H. Di, and G. AlRegib, 2017, Multiscale fusion for seismic geometric attribute enhancement: 87th SEG Technical Program Extended Abstracts, 2310-2314. (Link) (PDF) (Slides)
-
Huang, Y., and H. Di, 2017, Dip interpolation for improved multi-trace seismic attribute analysis: 87th SEG Technical Program Extended Abstracts, 2091-2095. (Link) (PDF)
-
Shafiq, M. A., Y. Alaudah, H. Di, and G. AlRegib, 2017, Salt dome detection within migrated seismic volumes using phase congruency: 87th SEG Technical Program Extended Abstracts, 2360-2365. (Link) (PDF) (Poster)
-
Di, H., M. Shafiq, and G. AlRegib, 2017, Multi-attribute k-means cluster analysis for salt boundary detection: 79th EAGE Conference and Exhibition, Tu-B4-09. (Link) (PDF)
-
Di, H., and G. AlRegib, 2017, Seismic multi-attribute classification for salt boundary detection: A comparison: 79th EAGE Conference and Exhibition, Tu-B4-13. (Link) (PDF)
-
Alaudah, Y., H. Di, and G. AlRegib, Weakly supervised seismic structure labelling via orthogonal non-negative matrix factorization: 79th EAGE Conference and Exhibition, Tu-B4-15. (Link) (PDF)
-
Alfarrj, M., H. Di, and G. AlRegib, 2017, Multiscale fusion for improved instantaneous attribute analysis: 79th EAGE Conference and Exhibition, Th-A4-13. (Link) (PDF)
-
Shafiq, M., H. Di, and G. AlRegib, 2017, A texture-based approach for automated detection of listric faults: 79th EAGE Conference and Exhibition, Th-P4-02. (Link) (PDF)
-
Di, H., and D. Gao, 2016, Improved seismic texture analysis based on non-linear gray-level transformation: 86th SEG Technical Program Expanded Abstracts, 2093-2097. (Link) (PDF)
-
Di, H., and D. Gao, 2015, Reflection geometry-based strain analysis from 3D seismic data: 85th SEG Technical Program Expanded Abstracts, 1922-1926. (Link) (PDF)
-
Di, H., and D. Gao, 2014, A new analytical method for azimuthal curvature analysis from 3D seismic data: 84th SEG Technical Program Expanded Abstracts, 1634-1638. (Link) (PDF)
-
Di, H., and D. Gao, 2014, Predicting fracture orientations with volumetric curvature gradient analysis: Case study from Teapot Dome in Wyoming: AAPG Annual Convention and Exhibition, Search and Discovery Article #41331. (Link) (PDF)
-
Di, H., and D. Gao, 2013, Gray-level transformation and Canny edge detection for 3D seismic discontinuity enhancement: 83rd SEG Technical Program Expanded Abstracts, 1504-1508. (Link) (PDF)
-
Bhattacharya, S., and H. Di, 2022, Advances in Subsurface Data Analytics: Traditional and Physics-Based Machine Learning, Elsevier (Edited book). (Link)
-
Di, H., and D. Gao, 2017, Seismic attribute-aided fault detection in petroleum industry: A review, in D. Martin (eds), Fault detection: Methods, Applications and Technology, 53-80. (Link) (PDF)

Books & Chapters

Dissertation & Thesis
May 2016
Seismic geometric attribute analysis for fracture characterization: New Methodologies and applications: Ph.D. Dissertation, West Virginia University. (Link) (PDF)
July 2011
分层均匀介质中有限震源引起的地面运动计算: 硕士论文, 中国地震局地球物理研究所 (Link) (PDF) Citation in English: Calculation of the ground motion caused by a finite source in stratified media: M.S. Thesis (in Chinese), Institute of Geophysics, China Earthquake Administration.